Key Takeaway
Predictive maintenance involves several key steps. First, collect data using sensors on equipment to monitor parameters like temperature, vibration, and pressure. This data collection is continuous, providing real-time insights into equipment health.
Next, use predictive analytics to analyze the collected data. Advanced algorithms detect patterns and anomalies that indicate potential issues. Implement condition monitoring tools to keep track of equipment status. Finally, develop implementation strategies to schedule maintenance before failures occur, minimizing downtime and repair costs.
Identifying Equipment for Predictive Maintenance
The first step in predictive maintenance is to identify which pieces of equipment are suitable for this type of maintenance. Not all machines may require predictive maintenance, and focusing on the most critical equipment can save time and resources. Start by looking at machines that are essential to your operation, those with a history of frequent breakdowns, or equipment that operates continuously.
For instance, in a factory with multiple production lines, you may notice that a particular machine, like a compressor or pump, tends to fail more often than others. Such equipment should be prioritized for predictive maintenance. By focusing on high-value or high-risk equipment, you’ll ensure the most significant return on investment. Identifying the right assets is key because predictive maintenance relies on gathering data from sensors, and installing them across every machine may not be practical.
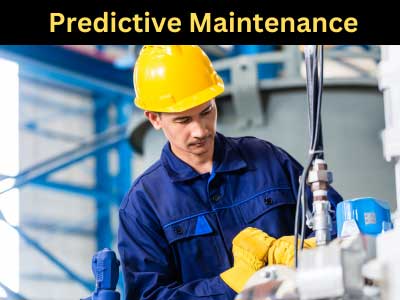
Setting Up Sensors and Condition Monitoring Systems
Once you’ve identified the equipment, the next step is to install sensors and set up condition monitoring systems. These sensors will track various parameters like temperature, vibration, pressure, and oil quality. Each type of sensor provides data that offers insight into how a machine is performing and whether it might be heading toward failure.
For example, vibration sensors can detect irregular movements in a motor that may suggest misalignment or bearing wear. Temperature sensors can alert you to overheating components, while oil analysis sensors monitor lubrication health, giving you early warning signs of contamination or degradation. By using a combination of these sensors, you can get a comprehensive view of each machine’s health.
Setting up condition monitoring systems isn’t a one-time task. The sensors must be calibrated correctly, and you should ensure that data from these sensors is flowing seamlessly to your monitoring software. This data will form the foundation for predicting when maintenance is needed. The goal is to capture small deviations from normal operation and flag them before they turn into larger problems. Condition monitoring systems act as the eyes and ears of predictive maintenance, constantly keeping an eye on the health of your equipment.
Collecting and Analyzing Equipment Data
Once the sensors are in place, the next critical step is data collection and analysis. Predictive maintenance heavily relies on real-time data to understand how a machine is operating. Over time, the sensors will gather thousands of data points on each piece of equipment, providing a clear picture of its health and performance trends. This wealth of data allows you to move from reactive maintenance (fixing things after they break) to predictive maintenance (fixing things before they fail).
Data analysis is where the magic happens. Specialized software processes the data and looks for patterns or anomalies that might indicate a developing problem. For instance, if a vibration sensor detects a sudden increase in vibration levels, this could suggest an imbalance or misalignment. By flagging this early, maintenance teams can schedule a repair before the issue worsens and causes an unexpected shutdown.
The analysis might also involve comparing current data to historical data, helping identify trends that lead to wear and tear over time. With the right tools in place, analyzing this data becomes more manageable, and the system can even suggest the most likely cause of the issue based on previous failures. Accurate data analysis is crucial in predicting the right time for maintenance, ensuring maximum uptime and minimal disruptions.
Implementing Predictive Maintenance Algorithms
The heart of predictive maintenance lies in the algorithms used to process and interpret the data collected from sensors. These algorithms utilize machine learning and artificial intelligence to detect patterns in equipment behavior that human operators might miss. Over time, they “learn” the normal operating conditions of each machine and can predict when deviations will lead to failures.
Implementing predictive maintenance algorithms requires a solid data infrastructure, as these algorithms need continuous data input to be effective. For example, an AI-powered algorithm might detect that a pump motor shows signs of increasing friction every time the machine exceeds a specific speed. The algorithm can then predict that this friction will cause a failure within a certain time frame if no maintenance is performed. Maintenance teams can intervene and replace worn parts well before a failure happens.
Additionally, these algorithms continuously improve as they receive more data. This means that the longer the system is in place, the more accurate its predictions become. However, it’s essential to periodically review the algorithms and adjust them if necessary to ensure they remain aligned with your equipment and operational needs. Advanced algorithms are the driving force behind the accuracy and efficiency of predictive maintenance strategies.
Continuous Monitoring and Optimization
Predictive maintenance is not a one-and-done process; it requires continuous monitoring and optimization to be truly effective. After implementing sensors, collecting data, and analyzing it, the work doesn’t stop. Continuous monitoring ensures that any small changes in equipment performance are identified early. This ongoing vigilance helps prevent surprises and ensures equipment remains in top condition.
Optimization is equally important. As you collect more data and fine-tune your predictive algorithms, you can start optimizing your maintenance schedules and resources. For example, instead of performing maintenance based on time intervals (like every six months), you can now perform maintenance only when needed, which is much more efficient and cost-effective. This shift from time-based to condition-based maintenance means fewer interruptions in operations and a more efficient use of resources.
Furthermore, continuous monitoring helps you refine your predictive maintenance strategy over time. The more data you collect, the better your understanding of each machine’s health. This process allows for incremental improvements, ensuring that your predictive maintenance program evolves with your operations. By keeping a close eye on equipment and continuously optimizing the maintenance approach, businesses can reduce downtime, cut costs, and improve overall productivity.
Conclusion
For predictive maintenance to succeed, adhering to best practices is crucial. The first step is identifying critical equipment that requires regular monitoring, especially those with a history of frequent failures or those vital to your operations. Installing the right sensors is the next step. These sensors track important parameters like vibration, temperature, and pressure to detect any abnormalities in equipment performance. Collecting accurate data is essential, as it provides the foundation for analyzing the health of the equipment. Data must be analyzed effectively to identify trends or deviations from normal operating conditions, which signal potential issues.
Continuous monitoring ensures that any developing problems are caught early before they lead to failures. This proactive approach helps optimize maintenance schedules and resources, preventing costly downtime and extending equipment lifespan. As more industries adopt predictive maintenance, a well-structured process helps businesses improve reliability, efficiency, and overall productivity, making maintenance more strategic and cost-effective.