Key Takeaway
Edge processing in IoT refers to processing data directly at or near the source of data generation, instead of sending it to a centralized cloud. It enables devices like sensors, cameras, or gateways to analyze and process data locally. This reduces the need for continuous data transfer, lowering latency and saving bandwidth. For instance, in a smart factory, edge processing can analyze machine data on-site for faster decision-making.
The key advantage of edge processing in IoT is real-time response. It’s especially useful in applications like autonomous vehicles, healthcare monitoring, and industrial automation, where every millisecond counts. By processing data locally, it improves security, as sensitive information doesn’t always need to travel over the internet. Edge processing is a cornerstone of IoT innovation, ensuring efficiency, speed, and scalability.
The Role of Edge Processing in IoT Applications
Edge processing is revolutionizing IoT applications by allowing real-time data analysis and decision-making at the device level. By processing data close to its source, IoT devices can operate independently and react immediately to changes in their environment. This role is especially critical in industries where timely actions are required, such as manufacturing, healthcare, and smart cities.
In a smart city, for instance, edge processing can manage traffic lights, monitor air quality, and adjust public lighting based on real-time data without the delay of communicating with the cloud. This leads to faster responses and improved efficiency in managing resources.
For industrial IoT (IIoT), edge processing enables predictive maintenance, where machines can analyze their own operational data and detect potential failures before they occur. By catching issues early, companies save on costly repairs and downtime. Additionally, edge processing allows for the monitoring and control of devices in remote locations where a reliable internet connection might not be available, like offshore oil rigs or remote factories.
Moreover, edge computing makes IoT applications more scalable. As more devices come online, cloud infrastructures can be overwhelmed by the sheer volume of data. By offloading data processing to the edge, only essential data is sent to the cloud, optimizing both bandwidth and storage. This distributed approach makes IoT systems more efficient and future-proof.
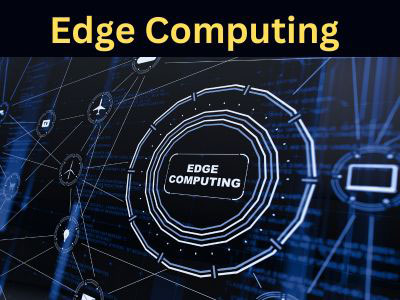
Benefits of Edge Computing for IoT Devices
Edge computing offers several key benefits to IoT devices that help optimize their performance, security, and overall efficiency. One of the most important advantages is reduced latency. By processing data locally, edge devices can make decisions in real time without waiting for data to travel to distant servers. This is critical for applications like autonomous vehicles, where split-second decisions can make the difference between safe operation and accidents.
Another significant benefit is improved security. Sending sensitive data to a centralized cloud server can expose it to potential cyber threats. With edge computing, data can be processed locally, reducing the risk of data breaches during transmission. Furthermore, encryption can be applied at the edge, enhancing privacy and securing sensitive IoT systems, especially in healthcare or financial industries.
Bandwidth optimization is another key benefit. In a typical IoT setup, millions of devices continuously generate data. Sending all this data to the cloud can consume vast amounts of bandwidth, increasing costs and slowing down network performance. Edge computing mitigates this by processing much of the data locally, only sending relevant, summarized data to the cloud. This helps in keeping the network load manageable and reduces the pressure on cloud storage systems.
Moreover, edge computing enhances reliability. In environments where constant connectivity might be an issue, such as rural areas or underdeveloped regions, edge devices can continue to operate autonomously without needing constant communication with the cloud. This makes IoT systems more resilient, especially in critical applications like remote monitoring, industrial control systems, and healthcare devices.
You May Like to Read
Challenges in Implementing Edge Processing in IoT
While edge processing brings numerous benefits to IoT systems, its implementation also comes with several challenges that need to be addressed for successful deployment. One major challenge is hardware limitations. Edge devices typically have less processing power, memory, and storage than cloud-based systems. This means that they are often unable to handle complex data analysis or process large datasets. In many cases, developers need to find ways to optimize algorithms and ensure that devices only process essential data without overloading their resources.
Another challenge is network infrastructure. While edge processing reduces the reliance on centralized servers, it still requires a reliable network to transmit data between devices and cloud systems when necessary. In remote locations or areas with weak connectivity, maintaining an effective network infrastructure can be difficult. Additionally, the growing number of IoT devices may lead to network congestion, impacting the efficiency of edge computing.
Security and data privacy are also significant concerns in edge processing. Since IoT devices are often deployed in unprotected or vulnerable environments, securing them against potential cyberattacks is critical. If an edge device is compromised, attackers may gain access to local data or control over other connected systems. Implementing robust security protocols and encryption at the edge is essential to safeguard data and protect against unauthorized access.
Finally, maintenance and scalability pose challenges as IoT systems grow. With multiple edge devices distributed across large areas, it can be difficult to manage and maintain them. Regular updates, troubleshooting, and ensuring scalability as the number of devices increases require careful planning and resources.
IoT Use Cases Benefiting from Edge Processing
Edge processing plays a vital role in enabling real-time responses in various IoT use cases, especially in industries that demand instant data processing. One prominent example is in healthcare. Remote patient monitoring, for instance, can benefit from edge processing by enabling devices like wearable ECG monitors to process and analyze data locally. In the case of irregularities in a patient’s heart rate, the device can immediately send an alert to the healthcare provider or take appropriate action, without waiting for cloud-based analysis.
In smart manufacturing, edge processing supports the concept of Industry 4.0 by enabling real-time monitoring of machinery and production lines. By analyzing data locally, machines can detect wear and tear, predict failures, and initiate maintenance processes without waiting for instructions from the cloud. This improves operational efficiency and reduces downtime, leading to cost savings.
Autonomous vehicles are another key area where edge computing is critical. These vehicles rely on sensors and cameras to collect data and make decisions in real-time. With edge processing, they can analyze the surrounding environment, detect obstacles, and make decisions without the need for cloud processing. This significantly reduces the time required for processing and enhances safety on the road.
In smart cities, edge computing enables the management of traffic systems, waste management, and even public safety. By processing data from sensors and cameras locally, traffic lights can adjust in real time to optimize traffic flow, while waste bins can signal when they are full, improving operational efficiency in urban areas.
Future Trends in IoT Edge Computing
The future of IoT edge computing looks incredibly promising as technology continues to evolve. One of the key trends we can expect to see is integration with 5G technology. With 5G’s high bandwidth and low latency, it will enhance the capabilities of edge processing, allowing even faster real-time data analysis and decision-making. This is particularly relevant for applications like augmented reality (AR), virtual reality (VR), and autonomous systems, which require ultra-low latency to function effectively.
Another trend is the growth of artificial intelligence (AI) at the edge. AI will enable devices to make smarter decisions without relying on cloud-based analysis. With advancements in machine learning and edge AI chips, devices will become increasingly autonomous, improving efficiency and providing deeper insights in areas like predictive maintenance, energy management, and personalized healthcare.
The rise of distributed edge networks is also something to watch. Instead of relying on a centralized cloud or edge server, distributed networks will involve a more decentralized approach, where smaller edge nodes work together to process data across vast geographical areas. This will improve scalability and reduce bottlenecks.
Additionally, security and privacy will continue to be a major focus. As more critical systems are moved to the edge, ensuring that data is protected against cyber threats will be paramount. Encryption, authentication, and robust security protocols will become even more important as edge devices become integral to industries such as healthcare, finance, and manufacturing.
Conclusion
In conclusion, edge processing is playing a pivotal role in transforming IoT applications. By enabling real-time data processing, reducing latency, and optimizing bandwidth, edge computing makes IoT devices smarter, more secure, and more efficient. While there are challenges, such as hardware limitations and network infrastructure, the benefits far outweigh the hurdles. As we move toward a more connected world, edge computing will continue to shape the future of IoT, creating new opportunities for industries and consumers alike.