Key Takeaway
Edge computing in AI refers to running artificial intelligence algorithms on edge devices, rather than sending data to the cloud. This allows AI to process data locally, reducing latency and improving response times.
For example, AI-powered cameras can recognize faces and make decisions in real-time without relying on cloud processing, enhancing applications like security and automation.
The Role of Edge Computing in AI Systems
Edge computing plays a critical role in enhancing AI systems by enabling real-time data processing at the point of origin. This reduces the need for sending large volumes of data to the cloud, thereby lowering latency and bandwidth usage. In AI applications like predictive maintenance or autonomous systems, edge computing allows immediate decision-making without cloud delays. It also improves data privacy by keeping sensitive information local, enhancing security. Edge computing is vital for AI systems that require fast processing, low latency, and efficient resource use, supporting smarter, faster, and more autonomous operations.
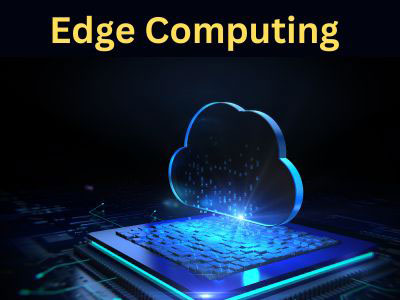
Benefits of Running AI Models at the Edge
Running AI models at the edge brings several advantages, particularly in terms of performance, efficiency, and security. Edge AI allows for local data processing, reducing the need to transmit data to the cloud, which can lower latency and reduce the risk of delays in critical decision-making processes.
One of the main benefits is faster decision-making. For applications like autonomous driving, healthcare monitoring, and industrial automation, it’s crucial that the system can process data quickly and make decisions in real-time. By running AI models locally on edge devices, the time needed for data transmission and cloud processing is minimized, which enhances the responsiveness of the system.
Data privacy and security are also significant advantages. With edge AI, sensitive data doesn’t need to leave the device, which reduces the risk of data breaches. For example, in healthcare applications, personal health data can be processed locally, without being transmitted to centralized servers, ensuring that privacy is maintained.
Another benefit is bandwidth conservation. Since edge devices process data locally, only relevant or summarized data needs to be sent to the cloud, which reduces network traffic and costs associated with transferring large amounts of raw data.
You May Like to Read
How Edge Computing Enhances AI for Real-Time Applications
Edge computing enhances the performance of artificial intelligence (AI) by enabling real-time data processing and decision-making directly at the source of data. In traditional cloud-based AI, data must be transmitted to remote servers for processing, which introduces significant latency and delays, especially in applications that require instant responses.
Edge computing reduces this latency by processing data locally on edge devices, allowing AI models to operate faster and more efficiently. For example, in autonomous vehicles, AI algorithms can analyze data from sensors, cameras, and LiDAR in real-time to make quick decisions regarding driving maneuvers, such as braking or steering, to avoid accidents. The ability to make these decisions instantly is critical for safety and performance.
In addition, edge computing allows for AI model updates and adaptation to changing conditions without needing to rely on constant cloud communications. Devices can be trained locally to optimize performance based on real-world data, reducing the time and resources required to send and receive data from remote servers.
Examples of Edge Computing Applied to AI Solutions
Edge computing plays a crucial role in AI applications by enabling real-time data processing and decision-making directly at the point of data generation. By leveraging edge devices, AI models can be deployed closer to the source of data, resulting in faster processing, reduced latency, and increased system efficiency. Several industries have begun adopting edge computing for AI applications, enhancing their capabilities and improving operational outcomes.
In the retail industry, edge computing is used to power AI-driven inventory management systems. Sensors and cameras installed in stores collect data on product availability and customer behaviors. Edge devices process this data in real-time, allowing retailers to adjust stock levels, optimize product placement, and enhance the customer experience without waiting for cloud-based analytics. This localized AI application not only improves decision-making but also reduces network congestion and delays in accessing cloud services.
Another prominent example is in the healthcare sector, where edge computing is used to power AI-based medical diagnostic tools. Edge devices in hospitals and clinics process patient data locally, enabling doctors to receive immediate insights from AI algorithms analyzing medical images, lab results, and patient histories. This reduces the dependency on cloud-based systems and ensures that critical medical decisions can be made quickly and accurately, improving patient care.
Overcoming Challenges in Edge AI Deployments
Deploying edge AI comes with its own set of challenges, including limited computational power. Edge devices typically have fewer resources than cloud servers, making it difficult to run complex AI models that require substantial processing power. To overcome this, developers often need to optimize AI algorithms and deploy lightweight models capable of running efficiently on edge devices without compromising performance.
Another challenge is ensuring data security and privacy. Since edge devices handle sensitive data locally, ensuring that this data is protected from breaches is essential. Encryption techniques, secure data storage, and real-time monitoring are necessary to safeguard data. Additionally, as edge AI often operates in distributed environments, ensuring that all devices comply with security protocols becomes more challenging. This requires the development of robust security frameworks tailored for edge computing environments.
Finally, managing the lifecycle of edge devices and AI models is a logistical challenge. Edge devices may be deployed in remote locations, making maintenance and updates difficult. Implementing over-the-air updates and centralized monitoring tools can help mitigate this challenge by enabling continuous monitoring and management of devices without requiring manual intervention. As edge AI continues to evolve, addressing these challenges will be key to its widespread adoption.
Conclusion
Edge computing in AI refers to the deployment of artificial intelligence models on edge devices that can process and analyze data locally, without sending it to the cloud for computation. This enables faster decision-making and reduces the bandwidth required for transmitting large datasets to the cloud. By integrating AI algorithms directly on edge devices, such as smartphones, drones, and industrial machines, edge computing empowers real-time applications like predictive maintenance, computer vision, and autonomous vehicles. This combination of AI and edge computing will drive the next wave of intelligent, responsive technologies.