Key Takeaway
The main drivers of industrial edge computing are the need for real-time data processing and reduced latency. In industrial environments, speed is crucial for making quick decisions, and edge computing allows data to be processed closer to its source, significantly reducing latency. This enables more efficient operations, faster response times, and improved productivity.
Another key driver is the desire for increased automation and control in industrial processes. Edge computing supports this by processing and analyzing data locally without relying on cloud-based systems. This reduces dependence on centralized systems, improves reliability, and makes it easier to scale operations across multiple devices and locations.
Demand for Real-Time Data Processing in Industries
In today’s fast-paced world, industries are relying more on real-time data processing to drive decision-making and enhance operational efficiency. Manufacturing plants, supply chains, healthcare systems, and logistics all require immediate access to up-to-date data to make quick and informed decisions. Traditional data processing models, which rely heavily on cloud systems, often introduce delays due to the time it takes to send data to distant servers and back. Real-time processing, however, enables businesses to analyze and respond to data as it is generated, allowing for faster actions and better performance outcomes.
This growing demand for real-time data processing is not just about speed but about accuracy and relevance. For example, in manufacturing, if a machine shows signs of failure, real-time data processing can detect the issue instantly, preventing costly downtime. Similarly, in healthcare, monitoring patients’ vital signs in real-time can save lives. This trend is driving industries to adopt edge computing, which brings data processing closer to the source. By doing so, businesses can minimize latency, ensure faster decision-making, and enhance overall operational efficiency.
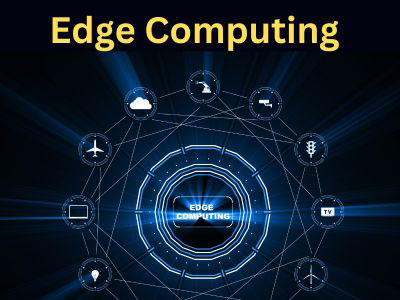
Adoption of Predictive Maintenance Solutions
Predictive maintenance has become a cornerstone of modern industrial operations. Unlike traditional maintenance, which relies on fixed schedules or reactive responses to equipment failures, predictive maintenance uses advanced technologies to forecast when a machine is likely to fail. This allows companies to address issues before they escalate, minimizing unplanned downtime and costly repairs.
Sensors embedded in industrial machinery continuously collect data such as temperature, vibration, and pressure. This data is then processed using machine learning algorithms that identify patterns and anomalies. For example, a slight increase in vibration might indicate bearing wear, prompting maintenance teams to take proactive action.
The benefits are clear—reduced operational costs, improved equipment lifespan, and enhanced safety. Industries like manufacturing, energy, and transportation are leading adopters of predictive maintenance solutions. For engineers, mastering tools such as IBM Maximo or GE’s Predix can be a game-changer. These platforms not only predict failures but also provide actionable insights to optimize performance.
As an engineer entering this space, understanding how to integrate IoT devices, manage data flow, and apply analytics is crucial. Predictive maintenance is not just about preventing breakdowns; it’s about fostering a culture of efficiency and reliability in industrial operations.
You May Like to Read
Need for Improved Operational Efficiency
Every industry aims to do more with less—less time, less energy, and fewer resources. Achieving this requires operational efficiency, which is one of the strongest drivers of industrial edge computing.
Edge computing streamlines processes by providing real-time insights and automating decisions. Consider a smart factory, where edge devices monitor and control machines. These devices can instantly adjust operations to maintain optimal performance. This ensures the factory produces high-quality goods while minimizing waste and energy consumption.
Another example is supply chain management. By using edge computing, companies can track goods in transit and respond to issues immediately. If a shipment is delayed, edge-powered systems can reroute other resources to avoid disruptions. This level of efficiency is impossible with slower, centralized systems.
Operational efficiency isn’t just about saving costs—it’s also about staying competitive. Companies that adopt edge computing can respond faster to market demands and customer needs. In today’s fast-paced world, this agility is a game-changer.
Simply put, edge computing empowers industries to be smarter, faster, and more efficient, helping them stay ahead of the competition.
Integration of IoT in Manufacturing Processes
The Internet of Things (IoT) is revolutionizing manufacturing. From smart sensors to connected machines, IoT devices are generating massive amounts of data. But managing this data effectively is a challenge. This is where edge computing makes a difference.
In manufacturing, IoT devices monitor production lines, detect anomalies, and optimize performance. However, sending all this data to the cloud for processing can cause delays. Edge computing eliminates this problem by processing data locally, right where it’s generated.
For example, in a car manufacturing plant, IoT sensors monitor assembly robots. If a robot’s performance deviates from the norm, edge devices can instantly detect the issue and adjust operations. This prevents defects and maintains production quality.
IoT and edge computing also enhance predictive analytics. By analyzing historical and real-time data, manufacturers can predict demand trends, optimize inventory, and improve supply chain efficiency.
Together, IoT and edge computing enable manufacturers to operate with precision and agility. This combination is driving the next wave of innovation in manufacturing, making processes smarter and more connected than ever before.
Growing Focus on Decentralized Data Analytics
Centralized data systems, where all information is sent to a single location for analysis, are no longer practical for industries handling massive amounts of data. Decentralized data analytics, enabled by edge computing, is becoming the norm.
With decentralized analytics, data is processed closer to where it’s generated. This not only reduces latency but also improves security by limiting the need to transfer sensitive data to external servers. In industries like healthcare, this is critical. Patient monitoring devices can analyze data locally, ensuring quick responses while maintaining privacy.
In manufacturing, decentralized analytics allow for better process control. For example, if a machine produces inconsistent outputs, edge devices can immediately analyze the data and make corrections without waiting for cloud-based systems.
Decentralized analytics also enable scalability. Industries can deploy edge devices across multiple locations, ensuring each site operates independently while contributing to overall efficiency. This approach reduces dependence on centralized systems, which can become bottlenecks as data volumes grow.
As industries focus more on data-driven decision-making, decentralized analytics powered by edge computing are unlocking new possibilities. It’s a trend that’s shaping the future of how businesses operate.
Conclusion
Industrial edge computing is no longer just a trend—it’s a necessity. By addressing challenges like real-time data processing, predictive maintenance, and operational efficiency, it’s transforming industries from the ground up.
For professionals entering the field, understanding these drivers is crucial. Industrial edge computing is not just about technology—it’s about solving real-world problems and creating value. As industries continue to embrace this technology, the future looks smarter, faster, and more efficient.