Key Takeaway
Edge AI faces several limitations that can impact its effectiveness. One major issue is resource constraints, as edge devices typically have limited processing power and storage compared to centralized systems. Additionally, the high costs associated with developing and deploying edge AI infrastructure can be a significant barrier for many organizations.
Integration challenges also arise, as ensuring compatibility with existing systems can be complex. Security risks remain a concern, with edge devices being vulnerable to both physical tampering and cyberattacks. Finally, scalability becomes an issue when managing a large number of edge devices across multiple locations. Despite these challenges, ongoing advancements continue to improve the efficiency and usability of edge AI.
Processing Power Constraints in Edge AI Devices
Edge AI devices are a key element in the evolution of edge computing, offering intelligent processing capabilities at the edge. However, despite their potential, processing power constraints remain a significant challenge for these devices. Unlike cloud-based systems, which have access to vast computational resources, edge AI devices must operate with limited power and processing capabilities, which can impact their performance.
One of the primary challenges is the size and power consumption of edge AI devices. These devices, often small and portable, are designed to operate in environments where space and power are limited. As a result, integrating powerful AI algorithms directly into these devices can be difficult, especially when considering the high computational requirements of modern machine learning models, such as deep learning or neural networks. These models require substantial processing power, which might not always be available on smaller, battery-powered devices.
To overcome these constraints, manufacturers are developing specialized AI chips and edge processors designed to be energy-efficient while still capable of running complex algorithms. Technologies like edge GPUs and ASICs (Application-Specific Integrated Circuits) are being used to optimize performance at the edge without draining power or increasing heat. These chips are specifically engineered to handle the high parallel processing required by AI tasks while minimizing power consumption.
Moreover, quantum computing and neuromorphic computing are emerging technologies that may help overcome these power limitations in the future. These innovative approaches to computing promise to deliver exponentially higher performance in edge AI devices, enabling them to process more data faster, without the constraints of traditional computing architectures.
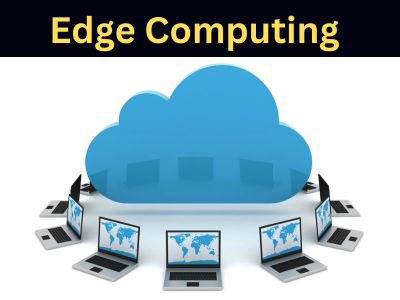
Security Challenges in Edge AI Deployments
As Edge AI becomes more widely adopted, security challenges are becoming increasingly important to address. Edge AI systems process sensitive data locally, which can introduce vulnerabilities if not properly secured. These challenges stem from the distributed nature of edge devices, the complexity of AI models, and the potential for unauthorized access.
One of the primary security concerns with Edge AI is data privacy. Edge devices often handle personal information, health data, or financial transactions, making them prime targets for cyberattacks. Without proper encryption and secure storage, attackers can gain access to this sensitive information, leading to data breaches and privacy violations. It’s essential for businesses to implement robust data protection measures to ensure the confidentiality and integrity of the data being processed at the edge.
Another major concern is AI model security. AI models used in edge devices are susceptible to adversarial attacks, where malicious actors manipulate input data to cause the model to make incorrect predictions or decisions. This could have disastrous consequences, particularly in high-stakes applications like autonomous vehicles or medical devices. Developing robust AI models that can detect and mitigate such attacks is crucial for securing Edge AI systems.
Device security is also a critical issue in edge AI deployments. Since edge devices are often deployed in remote or unsecured locations, they are vulnerable to physical tampering and unauthorized access. Protecting these devices with secure boot processes, tamper-resistant hardware, and regular firmware updates is vital to preventing attacks that could compromise the entire system.
You May Like to Read
High Costs Associated with Edge AI Infrastructure
Deploying Edge AI solutions can come with high upfront and ongoing costs. The need for specialized hardware, such as powerful processors, sensors, and communication modules, increases capital expenditures. Additionally, managing and maintaining a large network of distributed devices often incurs operational costs, including software updates, troubleshooting, and device replacements. Organizations must also account for the costs of integrating Edge AI into existing systems, training staff, and scaling the infrastructure to meet the demands of AI applications. These costs can be a barrier to entry for smaller businesses or industries with limited budgets.
Scalability Issues in Expanding Edge AI Applications
Scaling Edge AI applications across large networks can be challenging. While edge computing provides localized processing, managing and updating a vast number of distributed devices can be complex and time-consuming. Each device must be individually configured, maintained, and updated, creating significant overhead for IT teams.
Additionally, as the volume of edge devices increases, so does the complexity of managing them. Coordinating data flows, ensuring synchronization, and maintaining consistent performance across all devices becomes increasingly difficult as applications scale.
Balancing Data Privacy with Performance in Edge AI
Edge AI offers significant advantages in terms of data privacy because it processes data locally, reducing the need for sensitive data to travel across networks. However, ensuring that privacy regulations are adhered to while maintaining high performance can be tricky. Privacy measures such as data anonymization and encryption may impact the computational efficiency of AI models. For example, applying stringent data protection protocols can lead to slower processing times or higher energy consumption, which might reduce the overall effectiveness of edge AI applications. Balancing the need for high performance with compliance to privacy regulations is an ongoing challenge.
Conclusion
While Edge AI holds tremendous potential, it faces several key limitations that must be overcome to fully realize its benefits. Processing power constraints, security concerns, high infrastructure costs, scalability challenges, and balancing data privacy with performance are all hurdles that need to be addressed. As technology evolves, improvements in hardware, security protocols, and software solutions will likely mitigate some of these limitations. By navigating these challenges, Edge AI can become a more integral part of the future of intelligent, decentralized computing.