Key Takeaway
AI is not edge computing, but it can be used with edge computing. AI processes large amounts of data, and when combined with edge computing, it enables real-time decision-making at the device level.
For example, AI-powered cameras can analyze images locally, without sending data to the cloud. This allows for faster processing and quicker reactions in applications like security or healthcare.
How AI and Edge Computing Work Together
Artificial Intelligence (AI) and edge computing complement each other in powerful ways. AI systems are capable of processing large amounts of data and learning from patterns to make intelligent decisions. When integrated with edge computing, AI can process data locally, allowing for quicker responses without having to send the data to a centralized cloud server.
This is especially beneficial in areas like security surveillance, where AI algorithms can analyze video footage in real-time to detect unusual activities or recognize faces. In autonomous vehicles, AI algorithms can process sensor data immediately, allowing for faster decision-making on the road. As AI continues to evolve, its collaboration with edge computing will be key in enabling more advanced, real-time applications across industries.
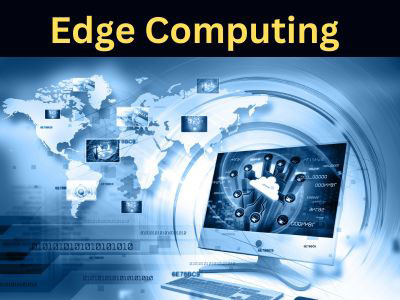
Benefits of Running AI on Edge Devices
Artificial Intelligence (AI) at the edge offers numerous benefits that elevate its utility across various industries. By processing data locally, edge devices reduce the need to communicate with the cloud. This not only brings faster response times but also decreases bandwidth usage.
Real-time analytics are possible as AI learns from data generated in real-time, making systems smarter and more responsive. For instance, retail environments can employ AI-enabled cameras for inventory management, analyzing stock levels without a round trip to the cloud.
As a new engineer, recognizing these efficiencies can inform how you design AI systems. Start considering which tasks could benefit from edge processing. This knowledge can guide project developments that harness AI’s full potential while optimizing performance.
By leveraging local processing, businesses can improve overall system functionality. This aligns with evolving demands for speed and adaptability in today’s technology landscape.
You May Like to Read
Key Differences Between AI and Edge Computing
The primary difference between AI and edge computing lies in their roles in data processing. AI requires vast computational resources and large datasets to train algorithms, often relying on cloud infrastructure to process this data. In contrast, edge computing processes data locally, at the “edge” of the network, which allows for faster decision-making and reduces the amount of data that needs to be sent to the cloud.
However, the two technologies often work together. Edge computing can serve as the environment for running AI models locally, especially in applications that require real-time processing, such as autonomous vehicles or industrial IoT. In these cases, edge computing reduces latency, while AI provides the intelligence to make decisions based on the data. While AI models can be heavy on computational needs, edge computing enables AI to operate more efficiently by processing data close to the source.
Examples of AI-Powered Edge Applications
AI-powered edge applications are revolutionizing industries by bringing the power of artificial intelligence directly to the devices where data is generated. Edge computing enables AI models to run locally on devices, allowing for faster, real-time decision-making without the need to transmit data to the cloud. This shift to AI at the edge enhances performance, reduces latency, and minimizes bandwidth costs, making it ideal for applications requiring immediate action.
In manufacturing, AI-powered edge solutions are used for predictive maintenance, where sensors on machines analyze operational data in real-time to detect signs of potential failure. By processing data at the edge, these systems can identify issues early, allowing for proactive repairs and minimizing downtime.
In healthcare, AI at the edge is used for medical imaging, where devices like MRI machines or diagnostic tools process images and provide real-time insights without relying on cloud servers. This helps healthcare professionals make faster, more accurate diagnoses.
Autonomous vehicles are another prime example of AI-powered edge applications. Self-driving cars use AI models to analyze data from sensors and cameras, making real-time decisions to navigate roads, avoid obstacles, and ensure passenger safety. By running AI models locally on the vehicle’s edge devices, the system can react instantly to changes in the environment, which is crucial for autonomous driving.
Challenges in Implementing AI on Edge Systems
Implementing AI on edge systems comes with several challenges that can limit its effectiveness and scalability. First and foremost, the computational power required to run advanced AI models can be too demanding for many edge devices, which are typically less powerful than cloud-based servers. Edge devices, especially in remote locations or those with limited resources, may struggle to process complex AI algorithms in real-time, potentially leading to delays or inaccuracies in decision-making.
Another challenge is the energy consumption of AI models. Running AI applications at the edge requires continuous power, and many edge devices are battery-powered, making it difficult to run resource-intensive models for extended periods without depleting their power source. As a result, companies must balance the power demands of AI with the limitations of edge devices.
Moreover, AI models often require large datasets to train, but the data generated at the edge is often limited. This makes it challenging to develop AI systems that can learn and improve without relying on centralized cloud processing. Additionally, edge devices must be capable of handling not only AI processing but also data storage, communication, and security, which can overwhelm the limited resources of many devices.
Conclusion
To conclude, AI and edge computing are two distinct but highly complementary technologies. While AI focuses on enabling machines to learn and make intelligent decisions, edge computing ensures that these AI processes occur locally, without relying on centralized cloud infrastructure. AI models can be deployed on edge devices to process data in real time, allowing for more responsive and autonomous systems. The integration of AI with edge computing enhances applications in various industries, such as healthcare, manufacturing, and transportation, by enabling quicker insights and reducing the reliance on cloud computing. Therefore, AI and edge computing work in tandem to drive innovation in many areas.