Key Takeaway
Yes, edge computing often uses AI to process and analyze data locally on devices. AI algorithms enable edge systems to make real-time decisions without relying on cloud-based servers.
This is critical for applications like autonomous vehicles, predictive maintenance, and smart cities. AI enhances edge computing by improving efficiency, reducing latency, and enabling intelligent actions at the source of data collection. This combination is key for modern technological advancements.
Understanding the Role of AI in Edge Computing
The role of AI (Artificial Intelligence) in Edge Computing is a game-changer for many industries, enabling intelligent decision-making at the device level. Traditionally, AI required powerful cloud servers to process vast amounts of data, but with the integration of AI into edge devices, data can now be processed locally, reducing latency and improving efficiency.
At its core, AI in edge computing allows devices to analyze data and make decisions without needing to send the information to a central server. For example, in autonomous vehicles, AI models process sensor data locally to make split-second decisions on the road. This is critical for ensuring safety and operational efficiency. Similarly, in industrial environments, AI models analyze data from sensors in real-time to detect equipment malfunctions, allowing for predictive maintenance and minimizing downtime.
One of the key benefits of integrating AI into edge devices is the ability to perform real-time data analysis. In sectors like healthcare, this means patient data can be analyzed on-site, allowing for quicker responses and better care without waiting for cloud processing. This also helps reduce the bandwidth required for transferring large data sets to the cloud.
Additionally, AI-driven edge devices are more capable of learning from the data they gather. They can adapt to new conditions and make informed decisions on the fly. This is particularly valuable in situations where continuous, real-time decisions need to be made, such as in smart cities, where AI models at the edge can adjust traffic lights, manage public utilities, or optimize energy use without waiting for cloud analysis.
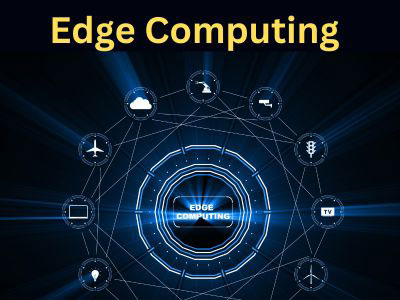
How AI Enhances Edge Computing Capabilities
The integration of artificial intelligence (AI) with edge computing significantly enhances the capabilities of edge devices, enabling them to make smarter, faster decisions in real-time. AI algorithms deployed at the edge allow devices to process and analyze data locally, without having to send it to the cloud. This reduces latency, improves operational efficiency, and enables more intelligent systems.
In autonomous vehicles, for example, AI at the edge enables vehicles to analyze sensor data, such as camera feeds, radar signals, and lidar inputs, and make immediate decisions about navigation and safety. By processing data on the vehicle itself, AI reduces the need for constant communication with the cloud, allowing for faster decision-making and safer driving.
In smart cities, AI-powered edge devices can monitor traffic patterns, air quality, and public safety in real-time. These devices can analyze data from sensors and cameras and make decisions such as adjusting traffic light timings, triggering alerts about pollution levels, or dispatching emergency services when needed. This real-time analysis allows cities to respond quickly to dynamic situations and optimize resources.
In industrial applications, AI at the edge is used for predictive maintenance. By analyzing data from machinery and equipment, edge devices can predict potential failures before they happen, reducing downtime and preventing costly repairs. AI also enables continuous performance monitoring and optimization of industrial processes, improving efficiency and reducing waste.
The combination of AI and edge computing is transforming industries by enabling devices to process complex data locally, make decisions autonomously, and take immediate actions. As more industries adopt these technologies, we can expect even more advanced applications that improve efficiency, safety, and customer experiences.
You May Like to Read
Applications of AI-Powered Edge Solutions
AI-powered edge solutions have revolutionized industries by bringing computation closer to the data source, enabling real-time decision-making and enhancing operational efficiency. In healthcare, AI at the edge helps with real-time patient monitoring and diagnostics, providing accurate results instantly and reducing dependency on cloud systems. In manufacturing, AI-driven edge computing facilitates predictive maintenance by analyzing sensor data from machinery, identifying potential failures before they occur, and optimizing production processes. Retailers benefit from AI at the edge by leveraging customer data to provide personalized experiences in real time, such as dynamic pricing and targeted promotions.
AI-powered edge solutions also enhance smart cities, where sensors and cameras process data locally, improving traffic management, monitoring air quality, and ensuring public safety. Additionally, autonomous vehicles are a major beneficiary, using edge AI to process sensor data instantly for navigation, object detection, and safety functions without the need for cloud processing. These applications highlight the importance of AI and edge computing working together to deliver faster, more accurate, and scalable solutions across various sectors.
Benefits of Using AI with Edge Computing Systems
The integration of Artificial Intelligence (AI) with Edge Computing systems brings numerous advantages to a wide range of industries. One of the main benefits is real-time data processing. Edge computing allows data to be processed locally, reducing latency and enabling AI models to make immediate decisions based on real-time inputs. This is critical in sectors such as healthcare, where AI can help with instant diagnostic decisions, or in autonomous vehicles, where split-second decisions can be the difference between safety and danger.
Another significant advantage is reduced bandwidth costs. By processing data at the edge, AI systems eliminate the need to send large volumes of raw data to the cloud, thereby saving on bandwidth and storage costs. This is particularly important in remote locations where internet access may be limited or expensive.
AI-powered edge devices are also more resilient and scalable. Since data is processed locally, edge systems are less dependent on cloud connectivity, ensuring continuous operations even in areas with intermittent internet access. This makes them ideal for applications in industries like manufacturing, where uptime is critical. Additionally, the scalability of AI on the edge means that systems can easily adapt to increased data loads or growing numbers of devices without requiring major infrastructure changes.
By combining AI and edge computing, businesses can also improve security. AI can detect and respond to threats in real time, reducing the risk of security breaches. This ability to combine intelligent decision-making with local processing makes AI-powered edge computing systems more powerful, flexible, and efficient across various applications.
Challenges in Integrating AI with Edge Technologies
Integrating AI with edge computing technologies presents several challenges that need to be addressed to unlock the full potential of both. One major issue is the limited processing power of edge devices compared to cloud-based systems. While edge computing enables real-time data processing at the source, these devices often have less computational power than traditional cloud infrastructures. As a result, training and running complex AI models at the edge can be resource-intensive, requiring edge devices to be more efficient or specialized in terms of hardware.
Another challenge is data synchronization and consistency. In many applications, edge devices must collaborate and share data to provide coherent insights. However, edge devices often operate in disconnected environments or with intermittent connectivity, making it difficult to ensure real-time synchronization across multiple devices. For AI applications that rely on large datasets, maintaining consistency across these edge devices while enabling machine learning algorithms to function correctly can be complex and resource-demanding.
Moreover, security concerns are heightened when integrating AI with edge technologies. AI systems on the edge process data locally, which increases the risk of data breaches if proper encryption and security protocols are not implemented. Ensuring that AI systems are secure while still providing real-time insights is a significant challenge that requires continuous monitoring and improvement.
Finally, scalability is a critical consideration when integrating AI with edge computing. As the number of edge devices increases, managing and optimizing AI models at scale becomes increasingly difficult. Ensuring these models perform well across a range of edge environments while maintaining high levels of accuracy and efficiency can be a logistical and technological hurdle for organizations.
Conclusion
In conclusion, Edge Computing and AI form a powerful combination that enhances real-time data processing and decision-making capabilities. While Edge Computing enables data to be processed closer to the source, reducing latency and reliance on cloud infrastructure, AI equips these systems with advanced decision-making power. This synergy enables faster and more intelligent applications, from autonomous vehicles to predictive maintenance in manufacturing. The integration of AI at the edge also improves operational efficiency and enhances customer experiences by enabling smarter and more responsive systems.
As Edge Computing continues to grow, AI’s role will become even more critical, empowering devices to make context-aware decisions and function autonomously. Industries such as healthcare, smart cities, and retail stand to benefit from AI-powered Edge solutions that can process and analyze vast amounts of data in real-time, ensuring better outcomes and improved performance. Together, Edge Computing and AI will continue to drive innovation across industries by providing high-speed, intelligent solutions that meet the demands of today’s data-driven world.