Key Takeaway
Deploying and managing AI at the edge comes with significant challenges. One of the primary issues is the complexity involved in setting up and maintaining edge AI systems. Additionally, edge devices often have limited processing power and storage capacity compared to centralized systems, which can restrict their performance.
Security is another concern, as edge AI devices, while keeping data local, remain vulnerable to physical tampering and cyberattacks. Integration with other systems can also be problematic, as seamless communication between edge AI and existing infrastructure is not always straightforward. Furthermore, the cost of implementing and maintaining edge AI solutions can be a barrier for many organizations.
Limitations of Edge Devices in AI Implementation
While edge computing combined with artificial intelligence (AI) offers incredible potential for real-time data processing and intelligent decision-making, there are certain limitations when it comes to implementing AI at the edge.
One of the key challenges is the computational power available at the edge. AI algorithms, especially deep learning models, require significant processing power and memory. Edge devices, which are typically smaller, lightweight, and power-efficient, may struggle to handle the heavy computational demands of complex AI tasks. As a result, edge devices may be limited in their ability to execute advanced AI algorithms, especially those that require high-speed data processing or complex neural networks.
Additionally, data storage is another limitation. While edge devices can process data locally, they have limited storage capacity compared to cloud servers or centralized data centers. AI applications often require large datasets for training models and improving decision-making. Storing and processing these large datasets directly on edge devices is not always feasible, especially in remote or resource-constrained environments.
Another challenge is data synchronization. AI at the edge often requires real-time data analysis, but it must also ensure that the data is consistent across different edge devices and the cloud. This can lead to synchronization issues, particularly when devices are spread across vast geographical areas or in environments with unreliable network connections.
Despite these limitations, advancements in edge AI hardware and software, such as specialized AI chips and edge-optimized machine learning frameworks, are helping to overcome these challenges. As edge computing and AI technologies continue to evolve, the limitations of edge devices in AI implementation are expected to be reduced, enabling even more powerful and intelligent edge applications in the future.
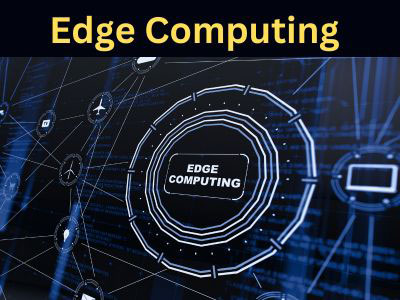
Security Risks Associated with Edge AI Applications
While edge computing offers many advantages, the integration of artificial intelligence (AI) at the edge introduces new security challenges that must be addressed to ensure the safety of sensitive data and systems. Edge AI applications involve processing data locally, often in remote or distributed environments, which creates opportunities for attackers to exploit vulnerabilities.
One major concern is data privacy. Since edge devices process data locally, they often handle sensitive information like personal data, health records, or financial transactions. If these devices are not properly secured, attackers can gain access to confidential data. Encryption and secure data storage are critical to mitigating this risk.
Another significant risk is device tampering. Many edge devices are deployed in unsecured or remote locations, making them more susceptible to physical attacks. Without proper safeguards, such as tamper-resistant hardware and secure boot mechanisms, edge devices can be compromised, allowing malicious actors to manipulate or steal data.
Moreover, AI algorithms used at the edge are vulnerable to adversarial attacks. In these attacks, malicious actors feed specially crafted input data into the AI system, causing it to make incorrect predictions or decisions. To prevent such attacks, it’s essential to build robust AI models that can detect and respond to adversarial inputs.
The decentralized nature of edge computing also creates challenges for network security. As data is processed and transmitted across a wide array of devices and networks, ensuring end-to-end security becomes more complex. Proper network segmentation, secure communication protocols, and real-time monitoring are necessary to safeguard the integrity of data as it moves between devices and central systems.
Challenges in Managing Edge AI Scalability
Scaling Edge AI solutions across multiple locations or devices can be complex.
Consistency Issues: Ensuring uniform model performance across varied edge devices is difficult.
Management Overhead: Updates, troubleshooting, and monitoring a distributed network of edge devices increase operational burdens.
Resource Allocation: Balancing computational loads across devices while avoiding resource bottlenecks is a major challenge.
Centralized management platforms and edge orchestration tools can help streamline scalability efforts, but these solutions are still evolving.
Latency and Connectivity Issues in Edge AI
While Edge AI aims to reduce latency, connectivity challenges can still create inefficiencies.
Network Dependency: Many edge AI systems rely on occasional cloud connectivity, which can result in delays during downtime.
Edge-to-Cloud Synchronization: Keeping data consistent between edge devices and central servers poses latency risks.
Bandwidth Limitations: High-bandwidth tasks, such as AI model updates, strain connectivity.
Reducing reliance on cloud servers and improving local processing capabilities are critical steps to overcoming these connectivity challenges.
Overcoming Barriers to Efficient Edge AI Deployment
Several strategies are emerging to address the challenges faced by Edge AI systems.
Model Optimization: Techniques like quantization and pruning make AI models lighter and more efficient for edge devices.
Improved Hardware: Specialized AI chips, such as edge TPUs, are enhancing computational power while maintaining energy efficiency.
Advanced Security Measures: Implementing AI-driven anomaly detection and encryption ensures better device protection.
Collaborative efforts between hardware manufacturers, software developers, and industries are critical for overcoming deployment barriers.
Conclusion
Edge AI presents unparalleled opportunities for real-time decision-making and localized intelligence, but challenges like hardware limitations, security risks, and scalability issues must be addressed. By leveraging advanced hardware, optimizing AI models, and enhancing security protocols, businesses can overcome these barriers.
The future of Edge AI lies in finding innovative solutions to these problems, enabling industries to unlock the true potential of this transformative technology.